Setting up the machine learning model for your segmentation strategy based on CLV.
In this post, we will guide you through setting up our powerful machine learning model to classify your customers by their CLV and unlock the potential of segmentation based on Customer Lifetime Value.
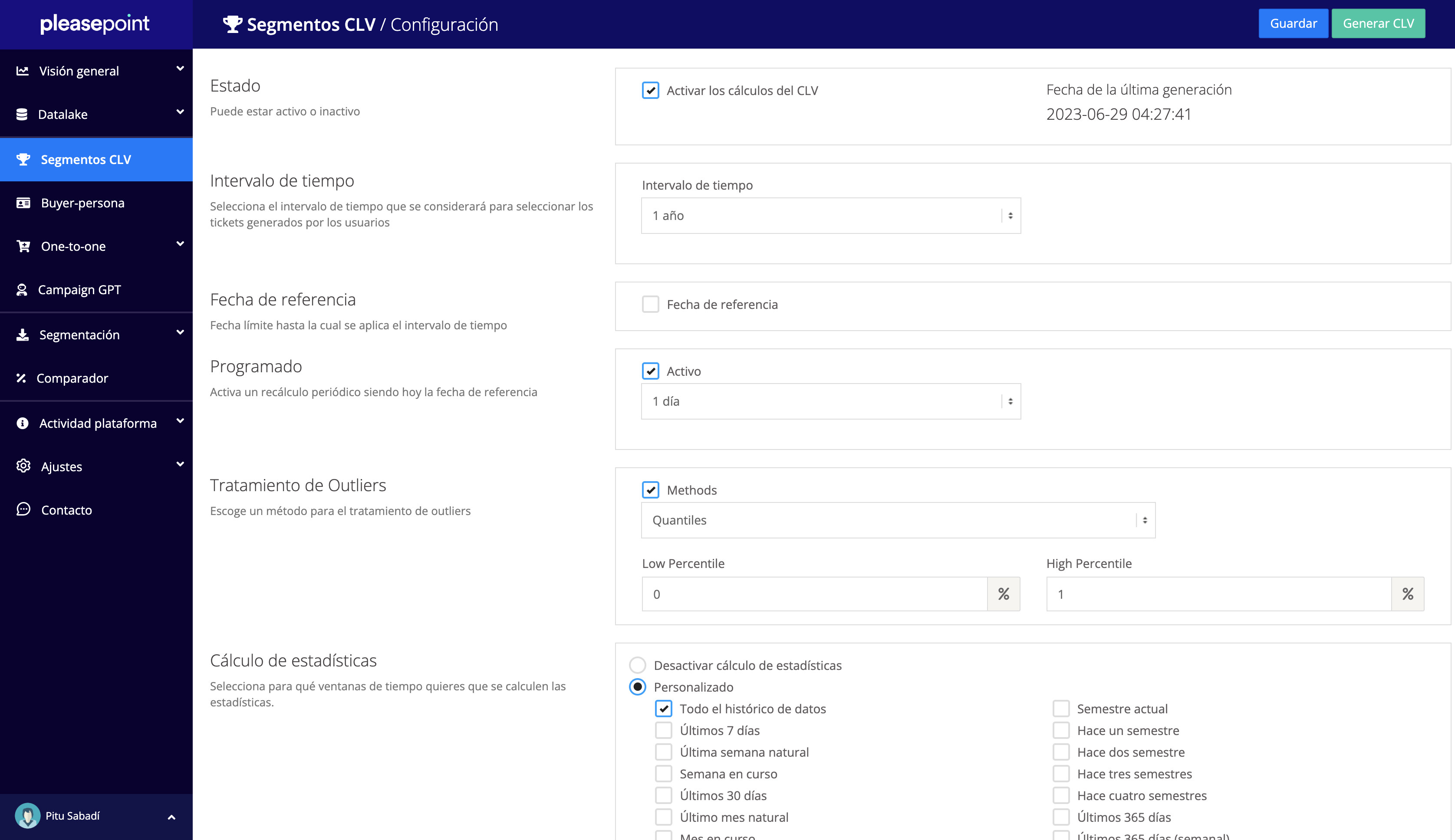
In the world of marketing, Customer Lifetime Value (CLV) plays a fundamental role. It is the indicator that allows us to understand the value each customer brings to our business throughout their lifecycle.
But how can we use this information to improve our marketing and personalization strategies?
In this post, we will guide you through the setup of our powerful machine learning model to classify your customers based on their CLV and unlock the potential of segmentation based on Customer Lifetime Value.
Segmentation based on Customer Lifetime Value provides you with a more precise and detailed view of your customers, allowing you to direct your marketing efforts more effectively.
By classifying your customers into different segments based on their CLV, you can tailor your messages, offers, and marketing actions to their specific needs and preferences.
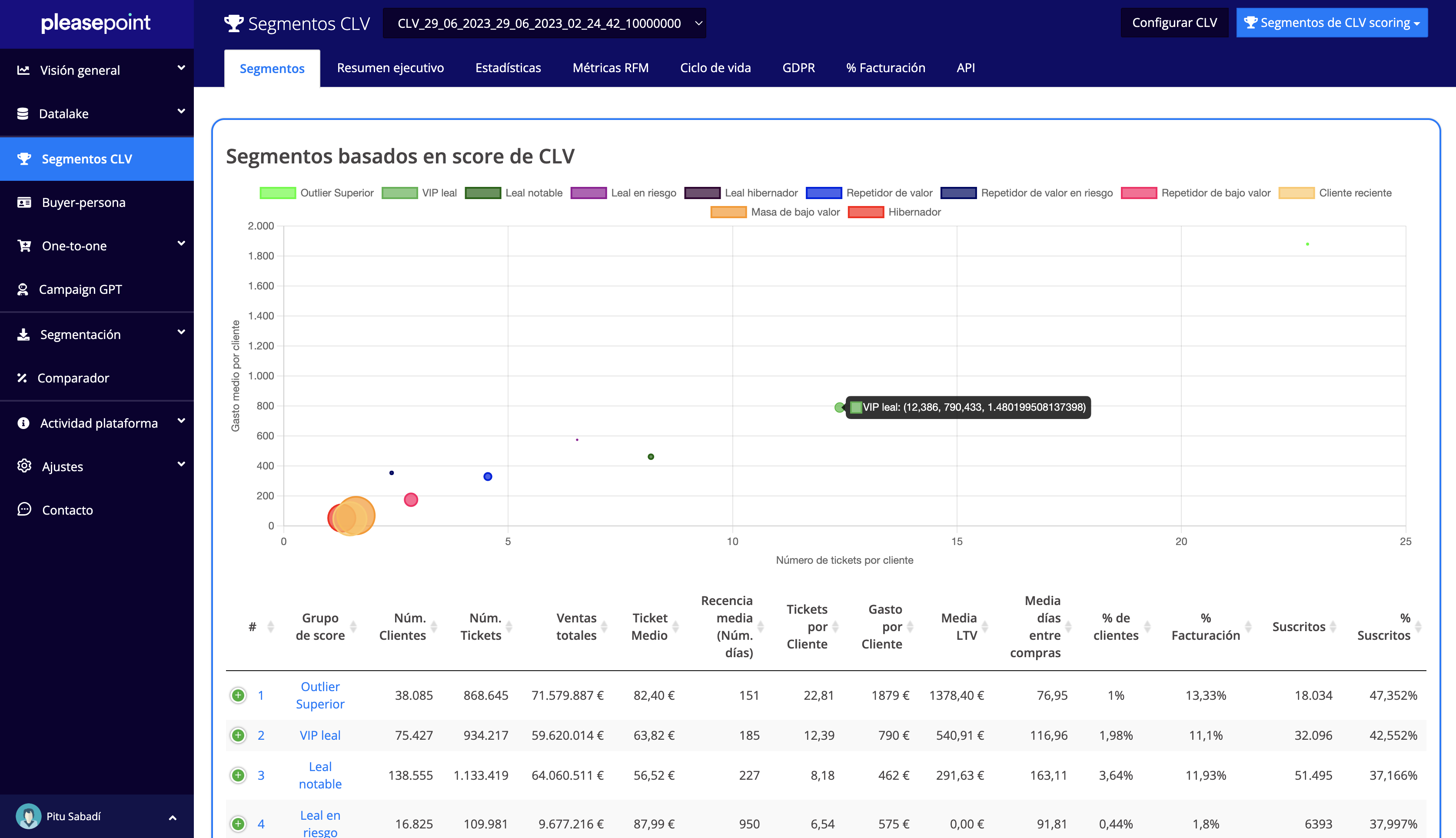
Imagine you have an online store that sells beauty products. Using our Customer Lifetime Value-based segmentation module, you can identify 12 key segments:
- Top outliers, the best customers with an unusual consumption pattern.
- Loyal VIP, your absolute best customers.
- Notable loyal, among your best customers without reaching the top.
- At-risk loyal, good customers at risk of being lost.
- Hibernating loyal, good customers you have already lost.
- Valuable repeater, customers in a healthy loyalty state with room for growth in their consumption.
- At-risk valuable repeater, good customers at risk of being lost.
- Low-value repeater, new customers starting to become loyal.
- Recent customer, new customer with the goal of encouraging repeat purchases.
- Low-value mass, customers you are struggling to retain—if not reactivated, they will become hibernators.
- Hibernators, customers who bought from you but you failed to retain.
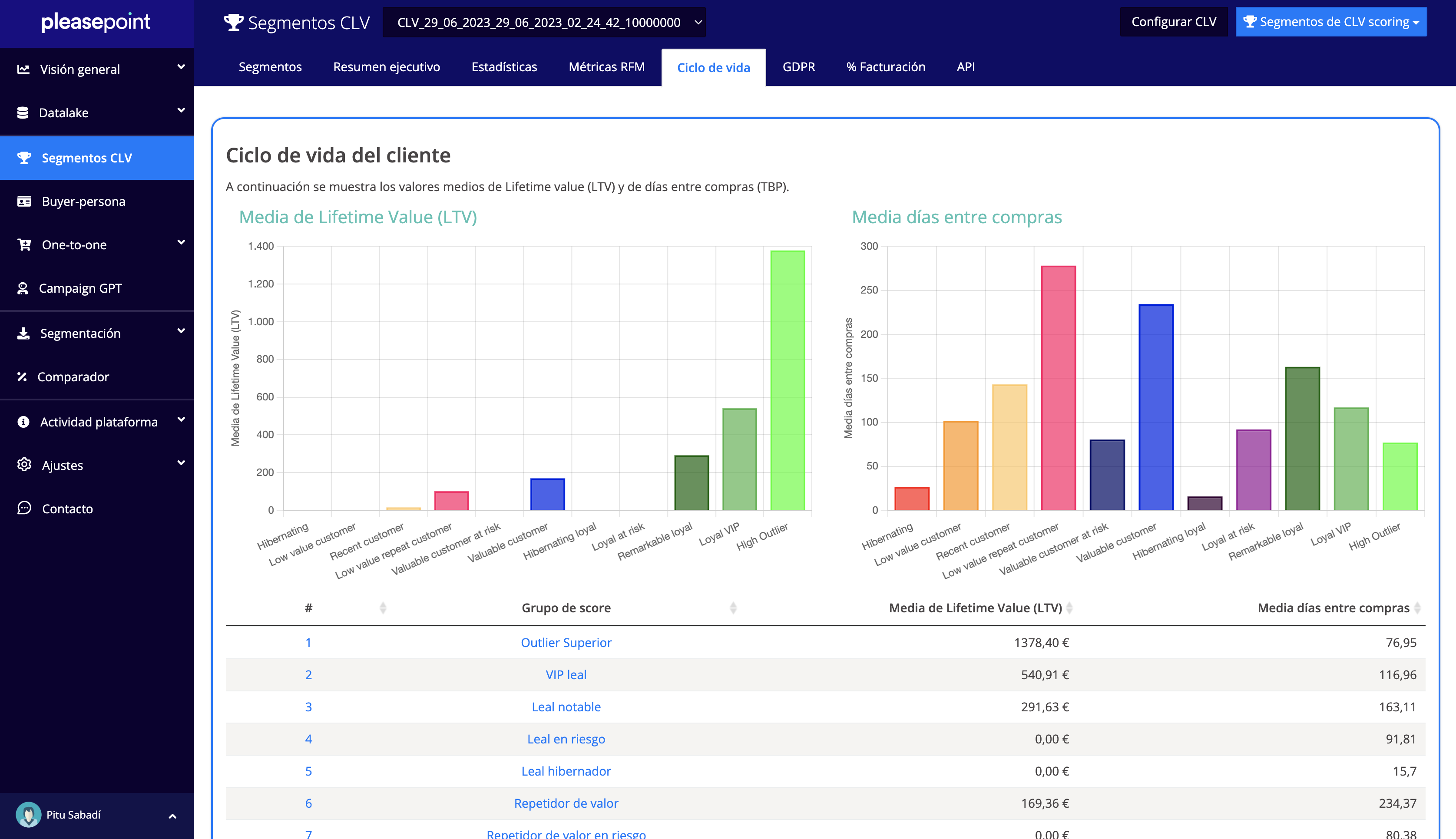
The first segments consist of your most loyal customers, those who have made multiple purchases in the past and continue to be loyal to your brand.
You can use this knowledge to thank them for their loyalty and reward them with exclusive offers or loyalty programs.
The middle segments are high-value customers, whose CLV is significantly higher than average. These customers deserve special attention, as they represent a significant part of your revenue.
You can design personalized campaigns for them, offering related products or premium services tailored to their needs and preferences.
And don't forget about the segments at risk of abandonment or hibernation. These customers have shown signs of inactivity or a decline in their CLV. This is where CLV-based segmentation comes into play. You can detect these customers and take action to rekindle their interest.
For example, you can send them persuasive messages, special discounts, or even offer them a personalized experience to win back their attention and loyalty.
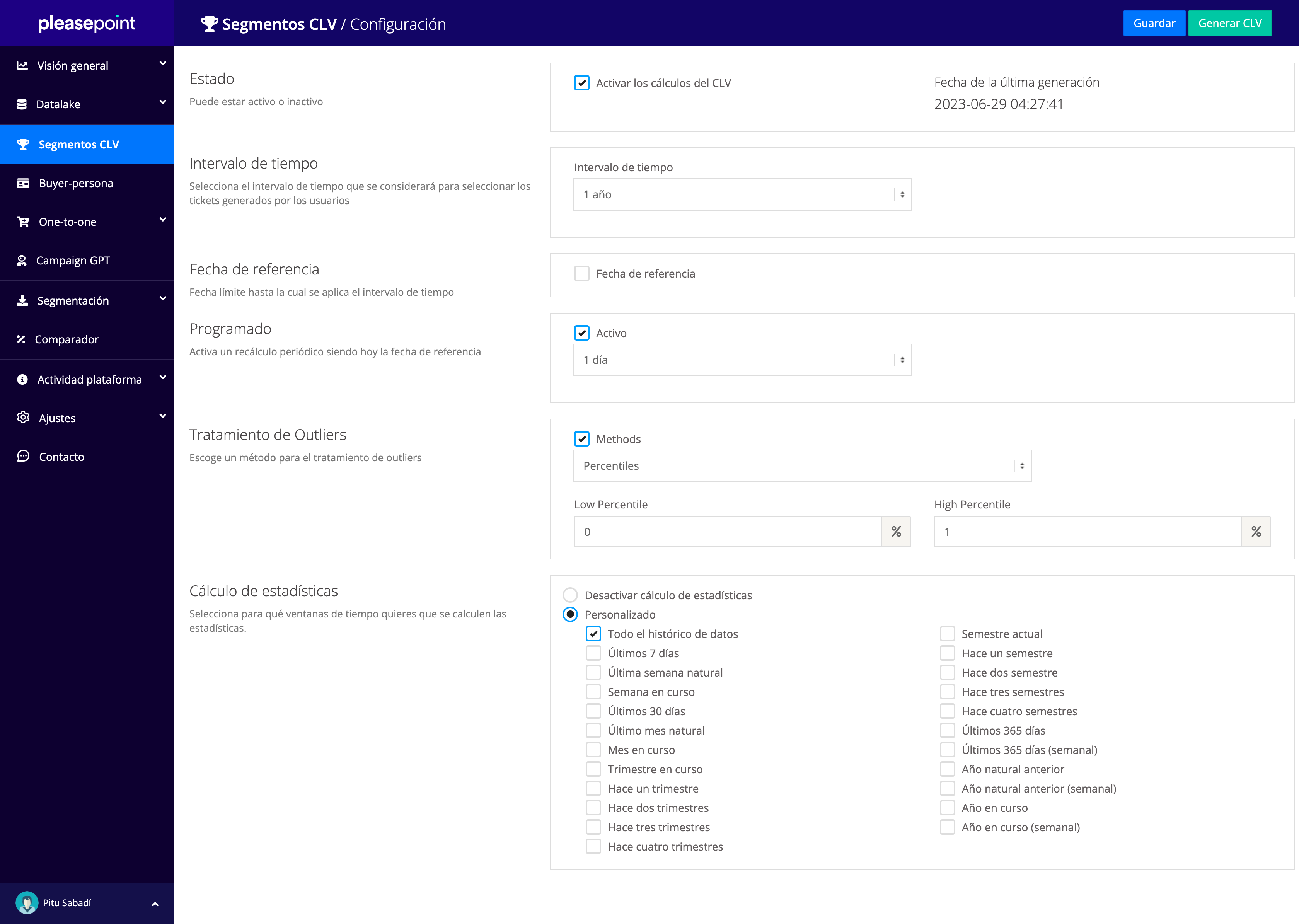
Now, let's talk about how to set up the machine learning model to achieve this precise segmentation.

First, you need to select the time interval you consider a customer to be inactive. This can vary depending on your industry and customer behavior.

Next, it's important to establish a data recalculation and customer classification strategy. This ensures your model is constantly updated with the latest information.

Additionally, you need to consider the treatment of outliers in your data. Outliers, which are atypical values, can distort the results of your model. Using techniques like percentiles or quantiles will help smooth out the effects of these outliers and achieve more accurate segmentation.
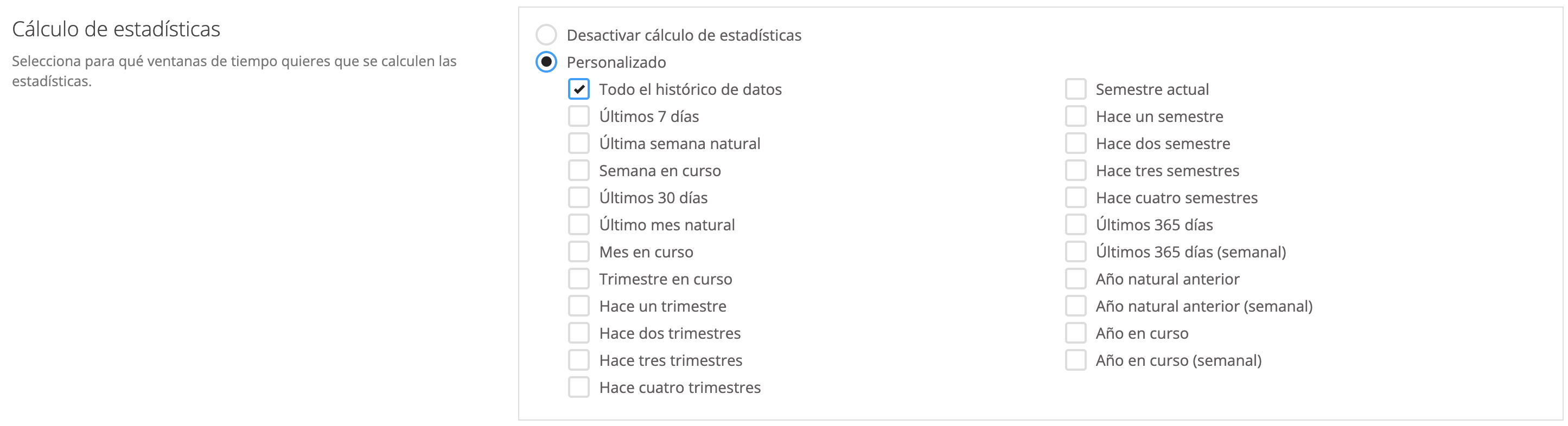
Finally, it is essential to establish appropriate statistical calculation windows. These windows define the time period during which statistical calculations are made to determine a customer's CLV.
Adjusting these windows will help you obtain more relevant results tailored to your business.
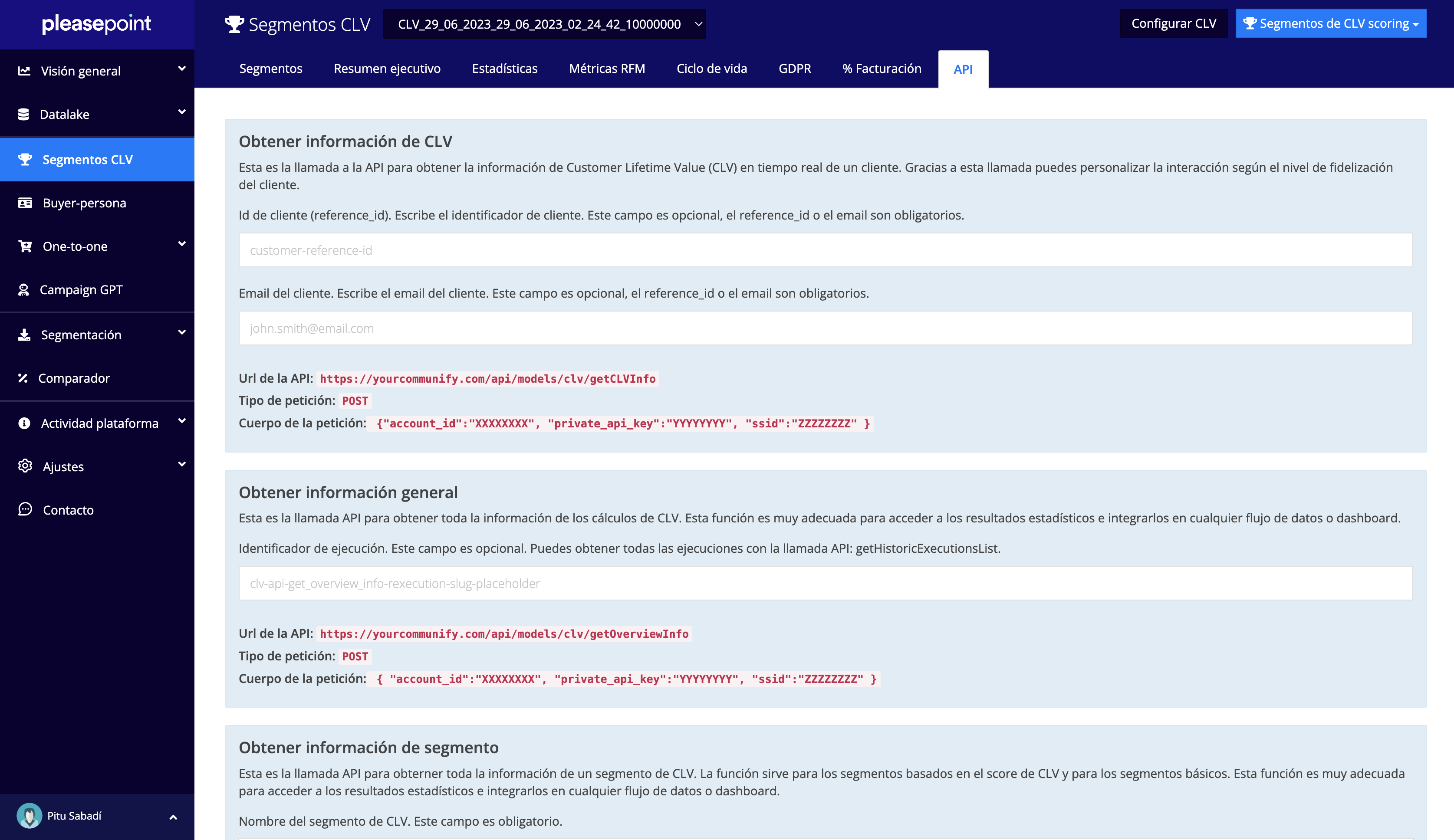
You can always access CLV information in real-time using our machine learning model API or having all this data enriched in your CRM, such as Salesforce Marketing Cloud.
In summary, segmentation based on Customer Lifetime Value is a powerful strategy for personalizing your marketing actions and optimizing your approach to each customer segment. Correctly configuring the machine learning model will allow you to make the most of this tool and improve your results.
Don't stop here.
Download our free one-to-one marketing ebook and discover more about how you can use Customer Lifetime Value-based segmentation to boost your personalized marketing strategy.
También te puede interesar...
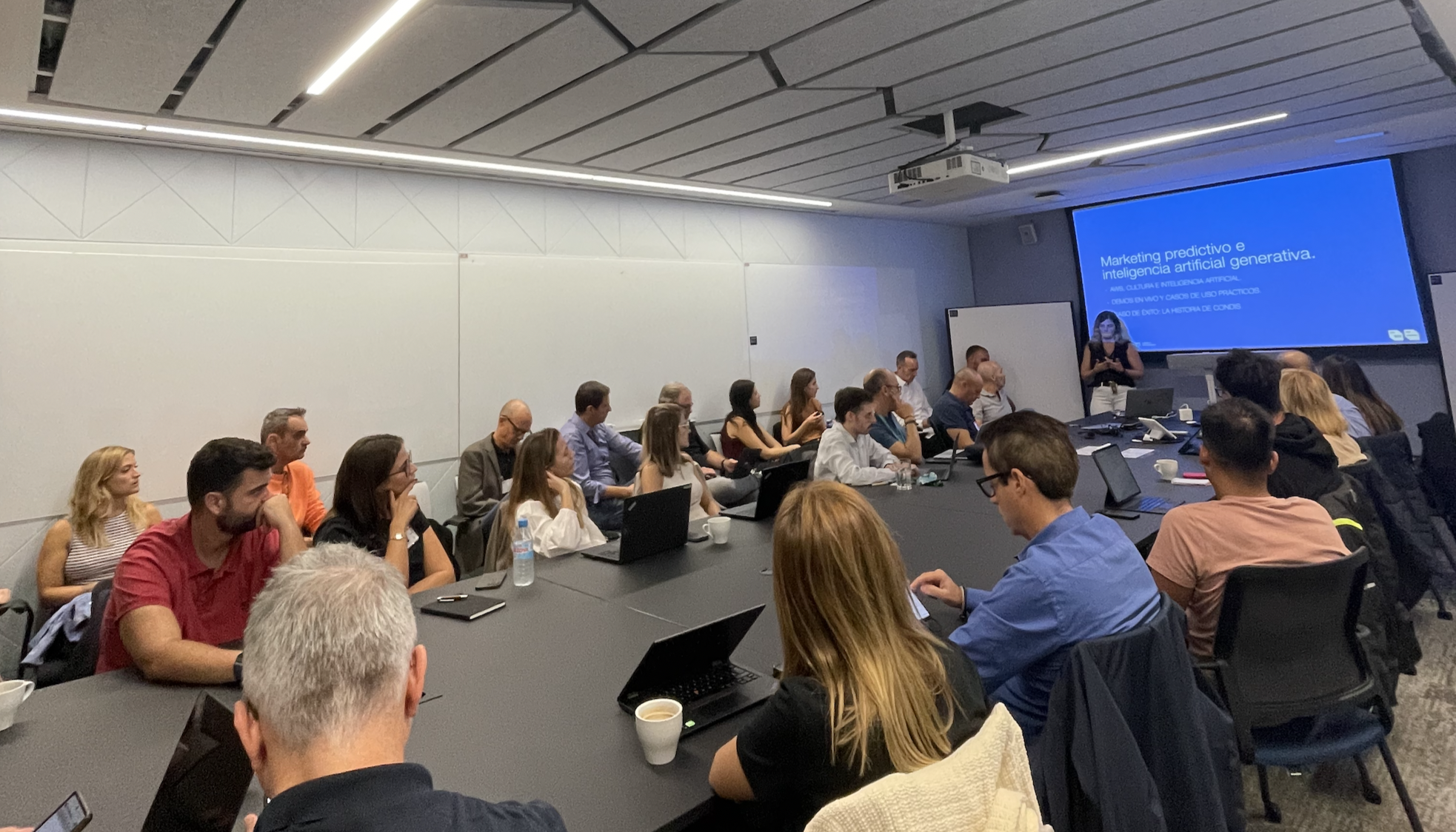
Event summary with Amazon Web Services in Barcelona.
We participated in the generative AI and predictive marketing session organized by AWS at their offices in Barcelona.
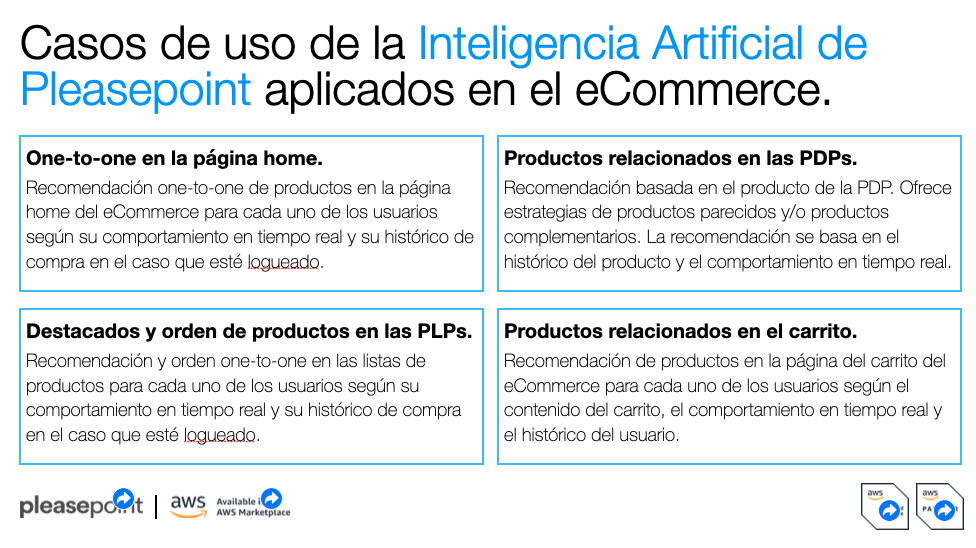
Five AI use cases applied in eCommerce.
We explore 5 AI use cases to show you how one-to-one personalization transforms interactions.
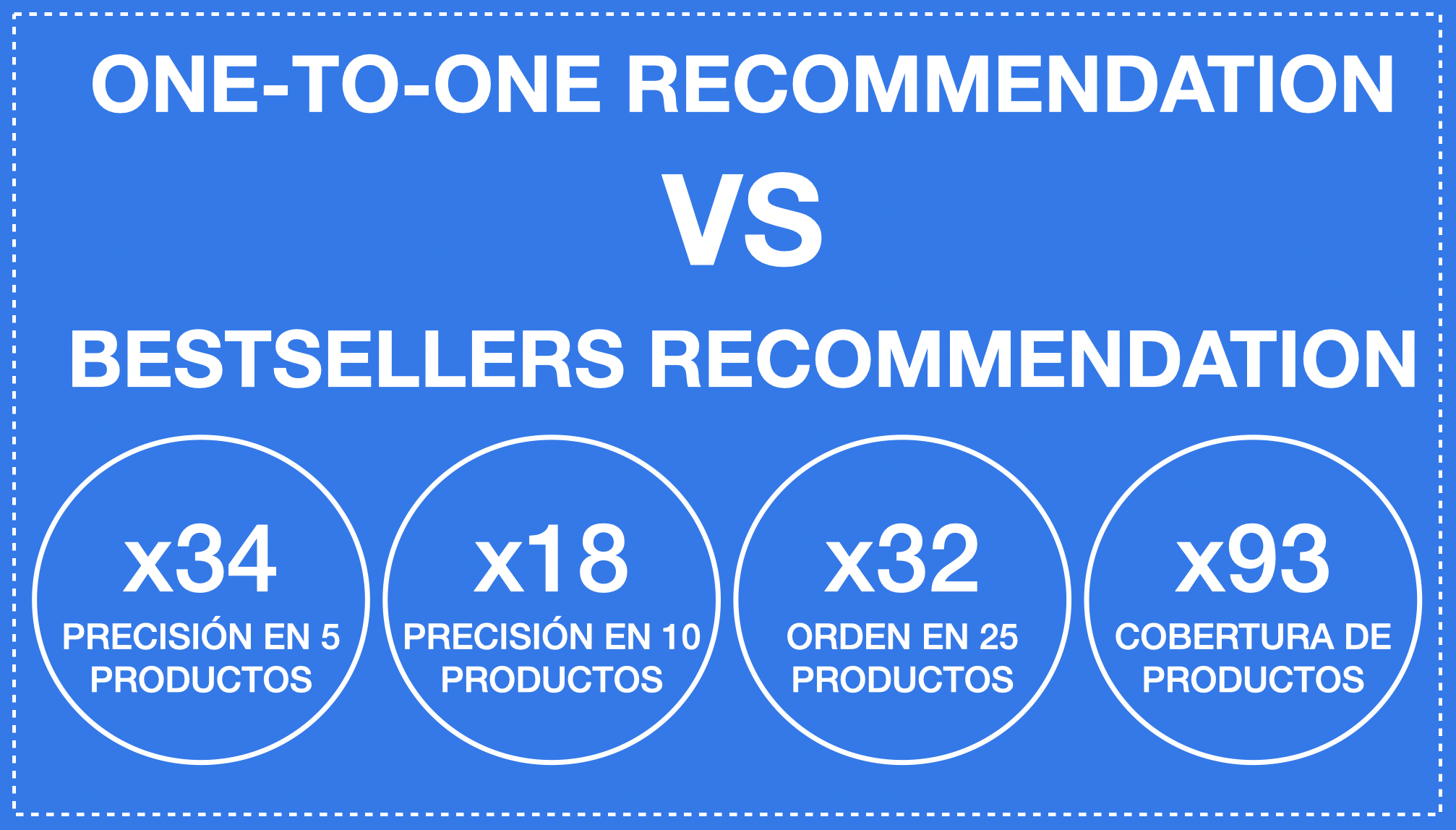
Benefits of one-to-one recommendations in eCommerce.
Benefits of one-to-one recommendations compared to traditional best-seller or trending product strategies.
Don't miss the upcoming Pleasepoint events!
Join our events and discover the best AI use cases. Learn from experts, participate in live demos, and access success stories that will drive your business.
Amazon Web Services (AWS) Software Partners (ISV).
We are ISV (Independent Software Vendor) partners of Amazon Web Services (AWS) and have been selected for the ISV-Accelerate program.
"With Pleasepoint, we have moved from the traditional campaign management model to a more dynamic, segmented, and enriched customer model, personalizing CRM campaigns and improving the conversion of our e-commerce channel customers."
Josep Jarque
Digital Project Manager & IT Architect"The implementation of one-to-one personalization has allowed us to generate much more interesting content for users, as well as foster loyalty, improve the shopping experience, and generate additional sales through personalized recommendations."
Jose Antonio Linde
COO of CondislineOur clients vouch for us.


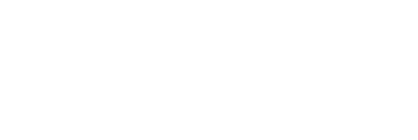
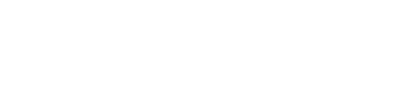
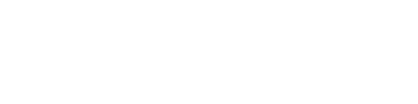
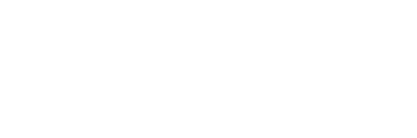
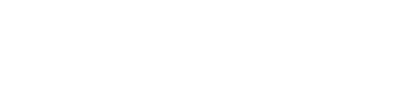
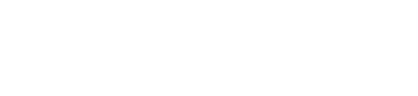
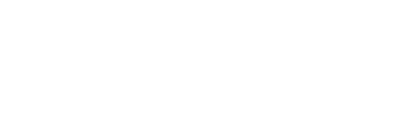

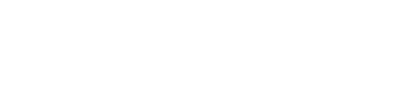
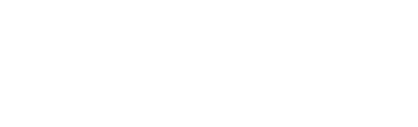