Benefits of real-time one-to-one product recommendations in eCommerce.
Benefits of Pleasepoint’s one-to-one recommendations compared to traditional strategies of displaying best-selling or trending products.
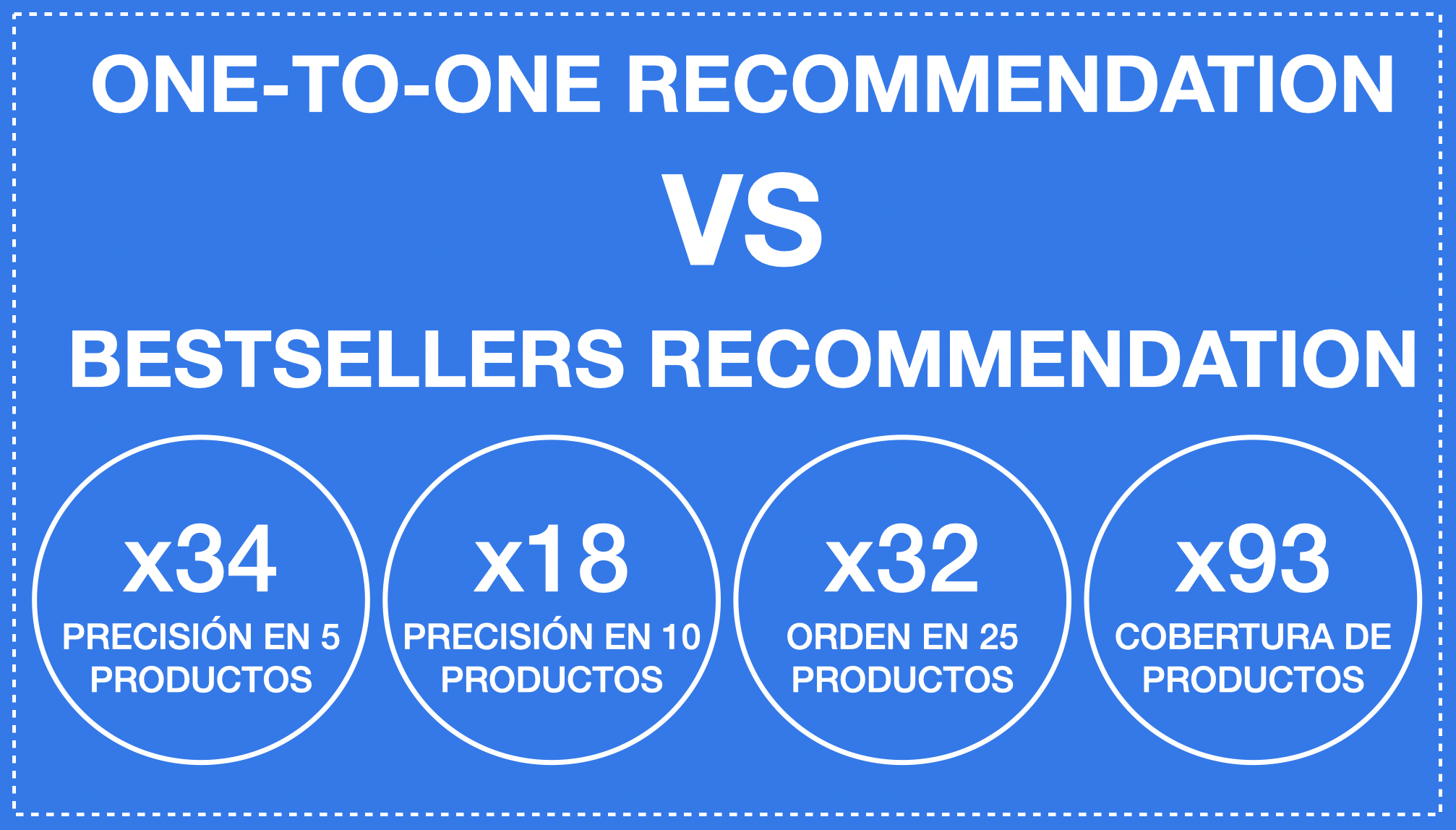
Personalization is a growing trend in the eCommerce world. Companies are looking to offer unique experiences to their customers, and one-to-one product recommendations are one of the most effective ways to achieve this.
In this post, we will explore the benefits of Pleasepoint's one-to-one recommendations compared to traditional strategies like showcasing best-selling or trending products. We will delve into how this technology, based on a neural network with reinforcement learning, can transform the way customers interact with your online store.
Here is the content of the article:
- Comparing one-to-one results with bestsellers.
- Detailed KPIs to assess the accuracy of the one-to-one recommendation model.
- One-to-one recommendation accuracy results.
- How to implement one-to-one recommendations step by step.
- Conclusions.
Pleasepoint's one-to-one recommendation system uses a neural network with reinforcement learning that feeds on both the user's purchase history and real-time browsing data. With the Pleasepoint neural network trained solely on your data, you will generate highly personalized product recommendations tailored to the specific preferences and behaviors of each customer.
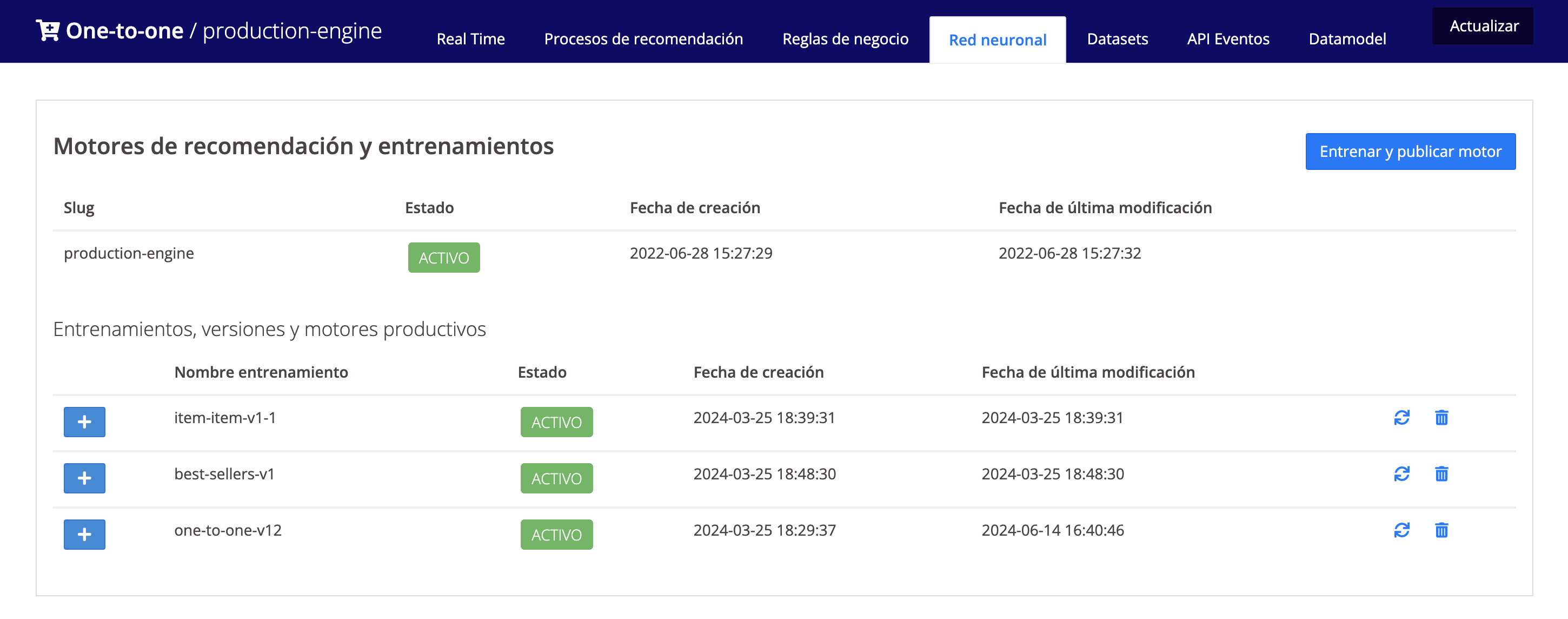
Reinforcement learning is an AI technique in which the model continuously learns from user interactions and improves its recommendations over time. This means that as more customers interact with your eCommerce, the accuracy and relevance of the product recommendations improve, providing an increasingly personalized and satisfying shopping experience.
Comparing one-to-one results with bestsellers.
To better understand the effectiveness of one-to-one recommendations, it’s useful to compare them with traditional strategies like recommending best-selling or trending products. While these strategies may seem effective, they tend to be generic and don’t always reflect each customer’s individual interests.
The comparison is done using several KPIs (key performance indicators) that allow us to measure the precision and effectiveness of both approaches to product recommendations. These KPIs take into account the accuracy of the recommendations, the accuracy in the order in which products are displayed, and the catalog coverage of products.
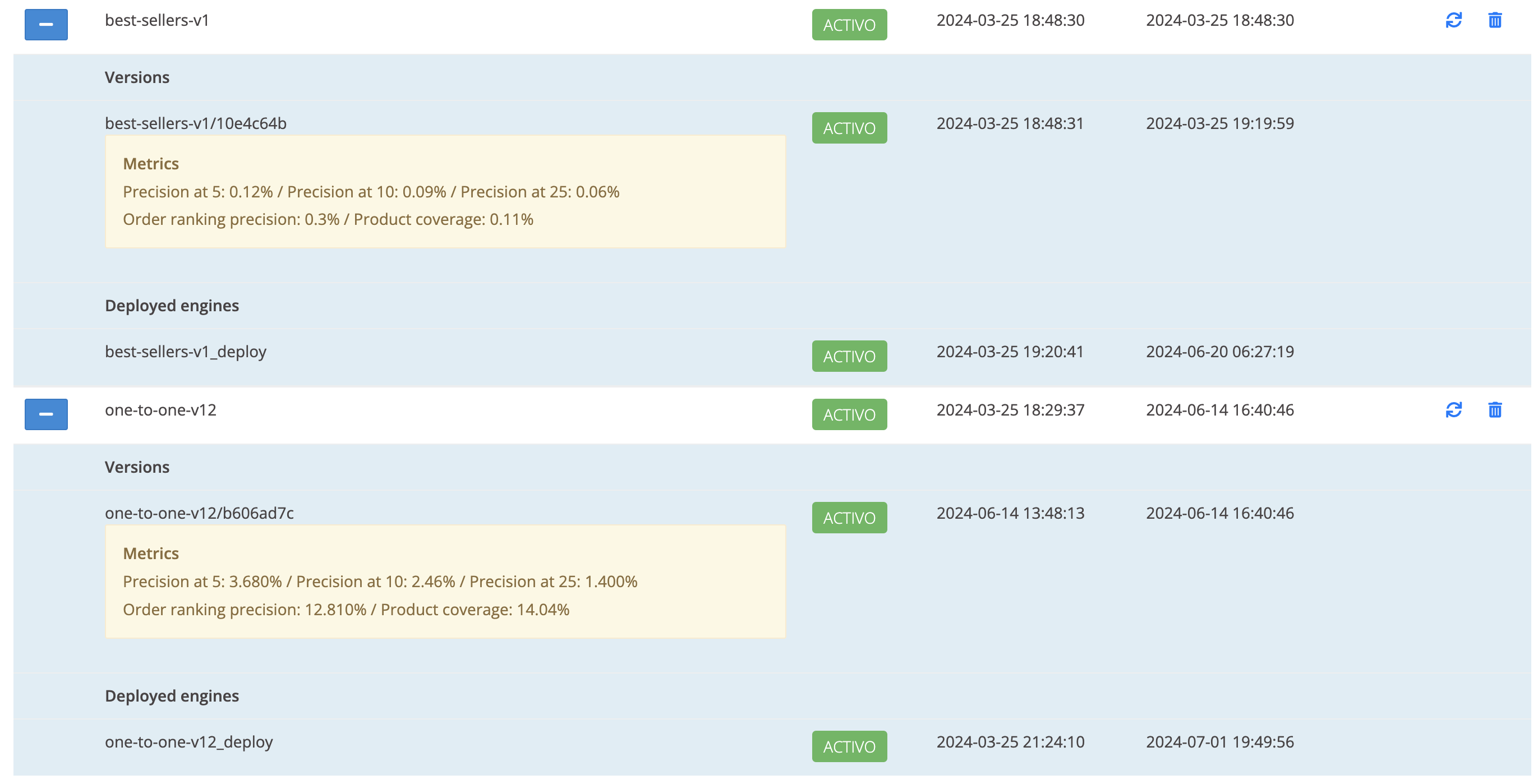
These indicators help quantify how much better one-to-one recommendations are in terms of relevance to the user and the diversity of products recommended during the real-time shopping experience in eCommerce.
Detailed KPIs to assess the accuracy of the one-to-one recommendation model.
To evaluate the quality of the one-to-one recommendation model, we will focus on different KPIs that allow us to assess the quality of the recommendations from various angles.
The first three focus on the quality of the recommendations using 5 products, 10 products, and 25 products. In these KPIs, we evaluate quality in terms of interaction and product purchase. The next KPI evaluates the quality of the order in which recommended products are displayed. The earlier a product is shown that the user interacts with and purchases, the better the KPI value.
Lastly, we will use the catalog coverage percentage to evaluate the diversity of products and the adaptability to each user’s preferences, tastes, and interests.
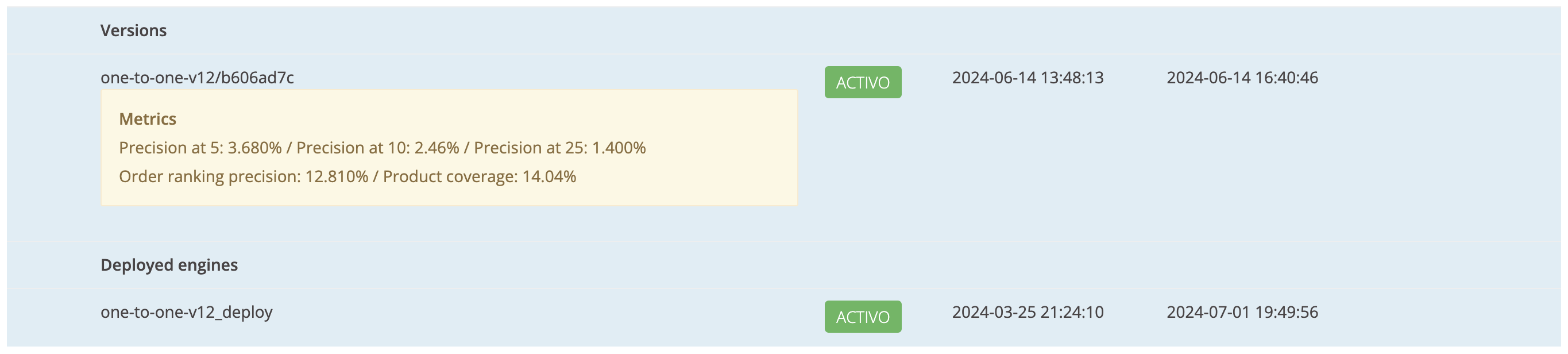
Here are the details of the KPIs:
- Accuracy at 5 products: Measures how often the top five recommended products are relevant to the user and lead to a purchase conversion.
- Accuracy at 10 products: Measures relevance within the top ten recommended products. This KPI uses the same logic as the previous one but expands the recommended product base.
- Accuracy at 25 products: Evaluates accuracy, similarly to the previous two cases, within a larger set of 25 recommended products.
- Accuracy in ordering 25 products: Not only measures if the products are relevant but also if they are optimally ordered according to user preference. For example, if the product that the user interacts with and purchases is in the first position, the KPI value is 100%; if it’s in the second position, it’s 50%; third position, 33%; fourth, 25%, and so on.
- Catalog coverage: Measures the diversity of recommended products, i.e., how many different products from the total catalog are recommended over time to each user.
One-to-one recommendation accuracy results.
To assess the accuracy of Pleasepoint's one-to-one recommendation, we use the calculated averages of the KPIs described for both the one-to-one recommendation model and the bestseller-based model.
These results were calculated using the average KPIs of all platform users, making it statistically significant. Remember, the one-to-one recommendation neural network is trained with your data and only your data.
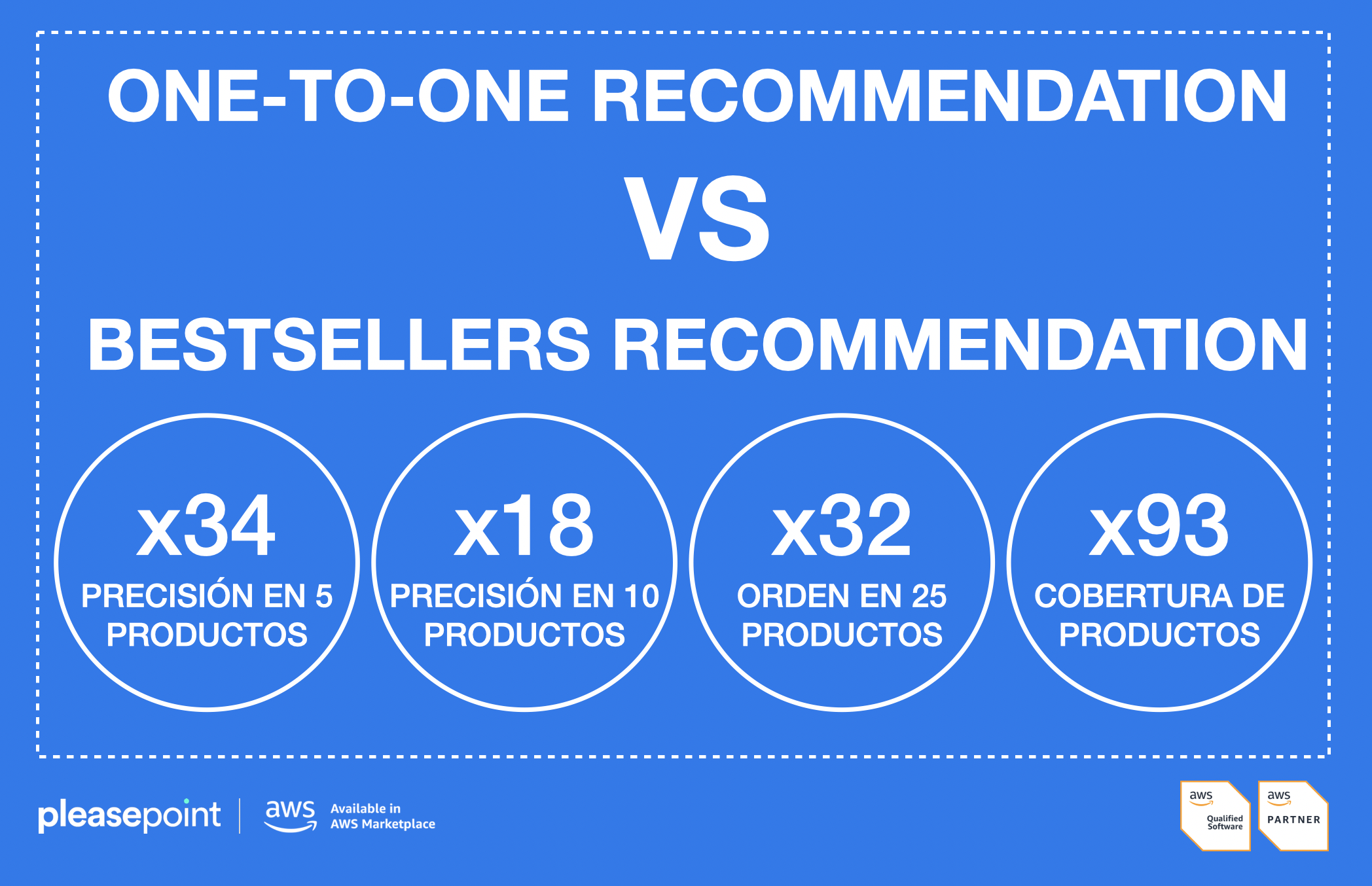
Here are the comparison results between the KPIs of the one-to-one recommendation and the bestseller recommendation strategy:
- Accuracy at 5 products: One-to-one recommendations showed a 34.45x improvement compared to bestsellers.
- Accuracy at 10 products: Accuracy improved by 18.76x with one-to-one recommendations.
- Accuracy at 25 products: The improvement was 11.09x in accuracy with one-to-one recommendations.
- Accuracy in ordering 25 products: The improvement was even more significant here, with a 32.13x increase in the accuracy of product ordering.
- Catalog coverage: One-to-one recommendations achieved a 93.88x improvement in catalog coverage, indicating greater diversity of recommended products.
These results demonstrate how one-to-one recommendations are more accurate and provide a broader and more diverse range of relevant products for each user.
How to implement one-to-one recommendations step by step.
Implementing one-to-one recommendations in your eCommerce is a structured process that we divide into four steps. Implementing a use case, such as one-to-one product recommendations on the home page, can be done in just a couple of days.
Here are the steps to implement one-to-one product recommendations in your eCommerce:
- Select a use case: Identify the areas of your eCommerce where personalization will have the most impact, such as the homepage, product pages, or the shopping cart.
- Upload historical data: Upload purchase and browsing history data to the Pleasepoint platform. This data is essential to train the recommendation model. Here’s the link to the documentation on the data model to work with.
- Register events: Set up real-time event tracking, such as product impressions, clicks, add-to-cart actions, and completed purchases. This allows the recommendation model to continuously update and improve thanks to reinforcement learning. This is the guide for the standard implementation of event tracking in an eCommerce.
- Display the recommendations: Integrate the Pleasepoint SDK into your eCommerce to display personalized recommendations on selected pages. Ensure that the recommendations are visible and easily accessible to maximize the use case impact. Here is the link to the complete SDK JS documentation for implementing one-to-one recommendations.
If you’d like more information about Pleasepoint’s JS and HTML SDKs for implementing one-to-one recommendations, you can access this link with complete usage information and SDK options.
Conclusions.
Pleasepoint's one-to-one recommendation offers a significant improvement compared to traditional bestseller strategies. Thanks to the neural network with reinforcement learning, it provides highly relevant and personalized product recommendations that increase customer satisfaction and conversion rates.
Implementing this technology in your eCommerce will not only improve your customers' experience but also boost your sales and customer loyalty. With the demonstrated KPI results and improvements in the article, it is clear that one-to-one recommendations are the way forward for any eCommerce seeking to stand out in a competitive market.
The journey to effective personalization starts with recognizing the unique needs of each customer, and implementing Pleasepoint efficiently meets those needs. With Pleasepoint, this journey is more accessible and rewarding than ever before.
También te puede interesar...
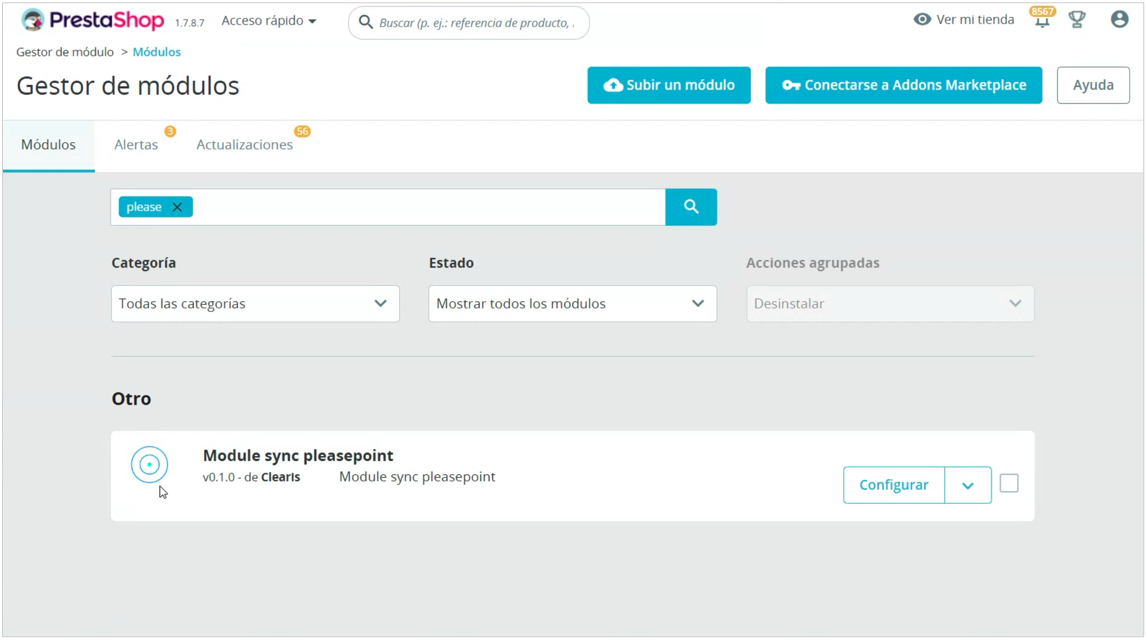
Launch of the Pleasepoint module for PrestaShop.
We make the ability to personalize more accessible, efficient, and effective with the Pleasepoint module for PrestaShop.
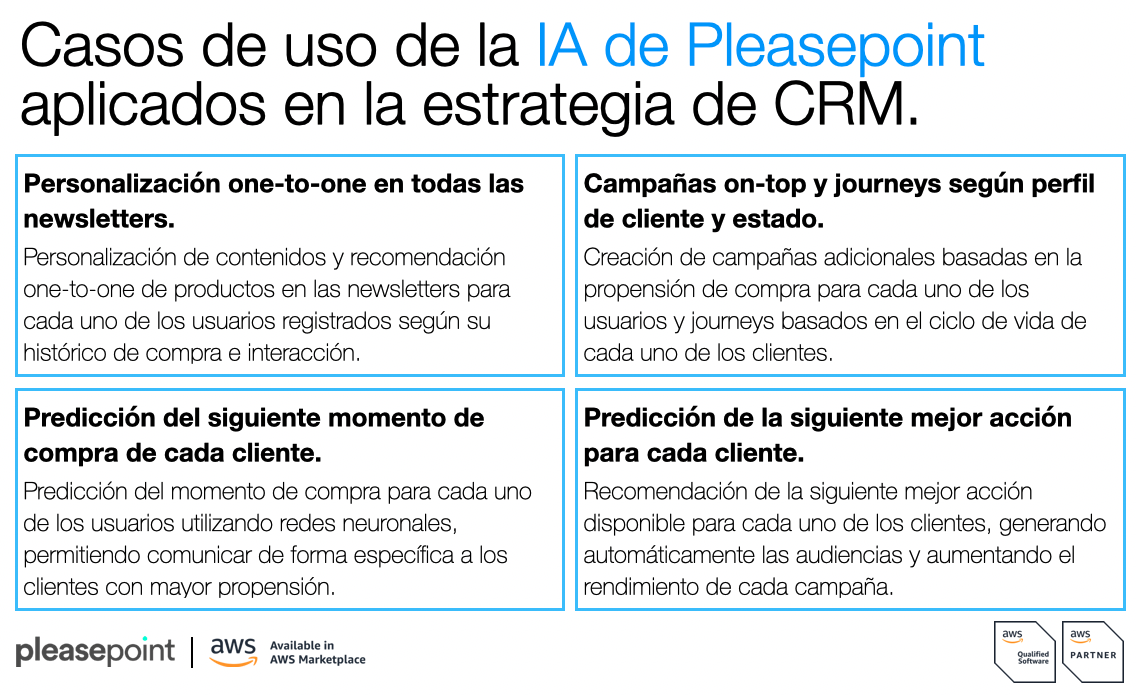
6 AI use cases applied in CRM.
We present 6 AI use cases for CRM communications personalization.
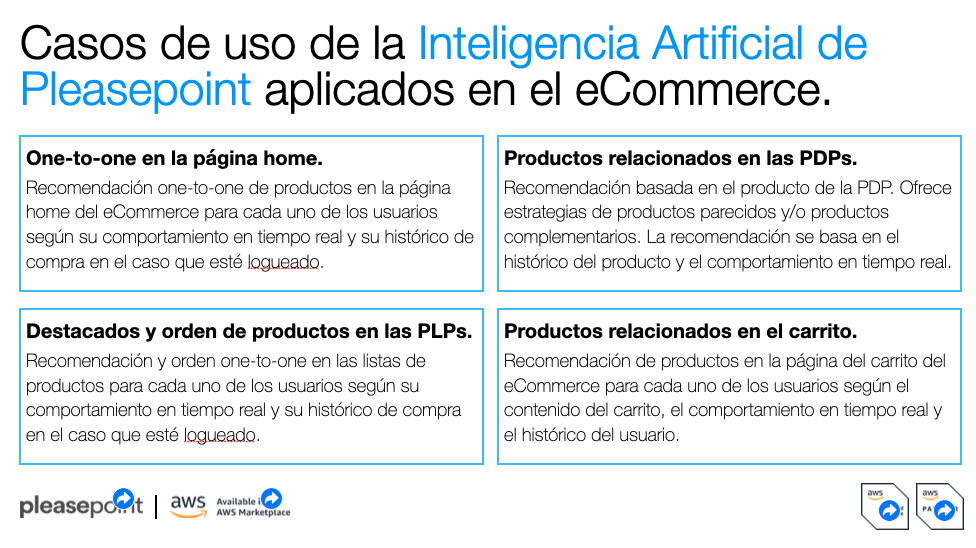
Five AI use cases applied in eCommerce.
We explore 5 AI use cases to show you how one-to-one personalization transforms interactions.
Don't miss the upcoming Pleasepoint events!
Join our events and discover the best AI use cases. Learn from experts, participate in live demos, and access success stories that will drive your business.
Amazon Web Services (AWS) Software Partners (ISV).
We are ISV (Independent Software Vendor) partners of Amazon Web Services (AWS) and have been selected for the ISV-Accelerate program.
"With Pleasepoint, we have moved from the traditional campaign management model to a more dynamic, segmented, and enriched customer model, personalizing CRM campaigns and improving the conversion of our e-commerce channel customers."
Josep Jarque
Digital Project Manager & IT Architect"The implementation of one-to-one personalization has allowed us to generate much more interesting content for users, as well as foster loyalty, improve the shopping experience, and generate additional sales through personalized recommendations."
Jose Antonio Linde
COO of CondislineOur clients vouch for us.


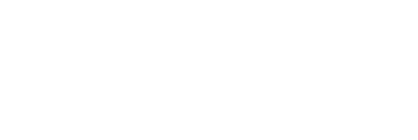
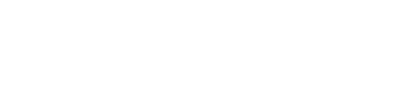
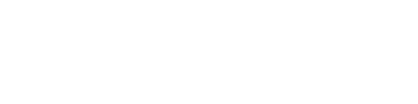
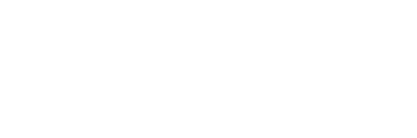
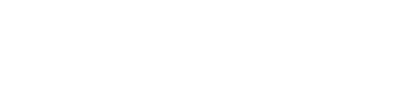
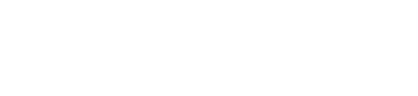
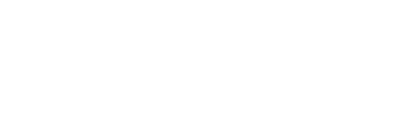

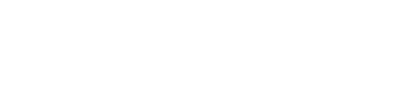
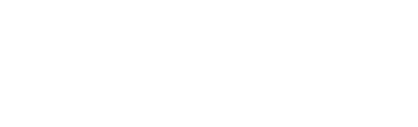